Artificial Intelligence in Diagnosis and Management of Ischemic Stroke
Brain Stroke is one of the major causes of disability and death worldwide. Approximately, 15 million people suffer from stroke every year and one-fourth strokes happen in people aged less than 65 years. Among the three types of stroke i.e. Ischemic Stroke (IS), hemorrhagic (bleeds) and transient ischemic attack (temporary clot), the IS accounts for about 85% of all stroke cases [1]. In stroke, the blood supply to the affected area in brain suddenly gets interrupted, and therefore, brain cells die quickly due to the lack of oxygen. The harmful effects of stroke vary with person to person and depend on the affected area in brain where the stroke happens, size of stroke, person’s general health and the most importantly, how quickly the patient received medical treatment. The delay in both diagnosis and treatment severely affects the brain function and increase the chances of mortality. As the brain controls all the physiological activities, a stroke may cause partial or complete paralysis, decreased sensation and perception, memory loss, behavior, and personality impairment. The seriousness of stroke can be understood by the fact that the only one-fourth of surviving individuals return to the normal health status. It is estimated that up to 80% of strokes can be prevented if one can identify or predict the occurrence of stroke in its early stage [2]. to estimate the probability of good functional outcome in post-stroke therapy. The prediction of functional outcome can be useful for patients, family members, and physicians, whose primary concern is not the likelihood of survival, but rather the likelihood of survival with the recovery of function [3]. Here, Artificial Intelligence (AI) offers technology solutions with high precision and accuracy for the diagnosis of stroke, its severity as well as prediction of functional outcomes. Recently, the U.S. Food and Drug Administration has approved the “Viz.AI Contact Application” to analyze Computed Tomography (CT) results that may notify physicians for a potential stroke in their patients. The Viz.AI Contact Application is a computer-aided software that uses an AI algorithm to analyze images for indicators associated with a stroke. It analyzes the CT images of brain and automatically identifies suspected Large-Vessel Occlusions (LVOs) and sends a text notification to the stroke physician within minutes. It is an important breakthrough toward using AI to make health care system more efficient and powerful [4,5].
AI is an area of computer science that aims to create intelligent machines that work and react like humans. It comprises the study and design of “Intelligent Systems” that receive the relevant information from the source and takes actions which maximize the chances of success. AI-assisted system works in three steps which include i. receiving information as “input data”, ii. data processing using a machine learning algorithm and iii. “Output Information” which is then used in decision making and action. The success of an AI system solely depends upon the authenticity of the input data i.e. the information and the applied machine learning protocol to develop AI system. AI has brought a paradigm shift to the healthcare system, driven by increasing availability of healthcare data and rapid progress of machine learning techniques such as Artificial Neural Networks and Deep Learning. In healthcare, AI is being used to mimic human cognitive function in the field such as psychology, neuroscience, cognitive science, speech and facial recognition (Table 1). Major disease area that uses AI techniques include cancer, neurology, endocrine, nutritional and cardiology (Figure 1) [6]. In recent years, several AI techniques have been used in several stroke-related cases to facilitate clinical decision and have shown AI’s potential in timely detection and treatment of stroke (Table 2). This is observed that AI can diagnose strokes and brain hemorrhage more accurately and faster than humans [7]. The present article focuses on the application of AI techniques in the stroke which includes early detection and diagnosis, treatment and outcome prediction.
Table 1: AI based applications for diagnosis and management of various diseases.
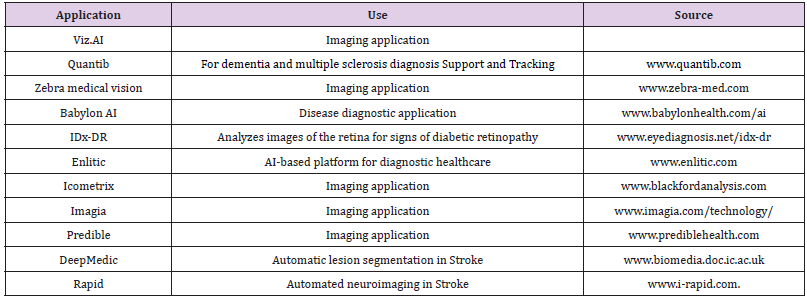
Table 2: Machine-learning studies on stroke imaging.
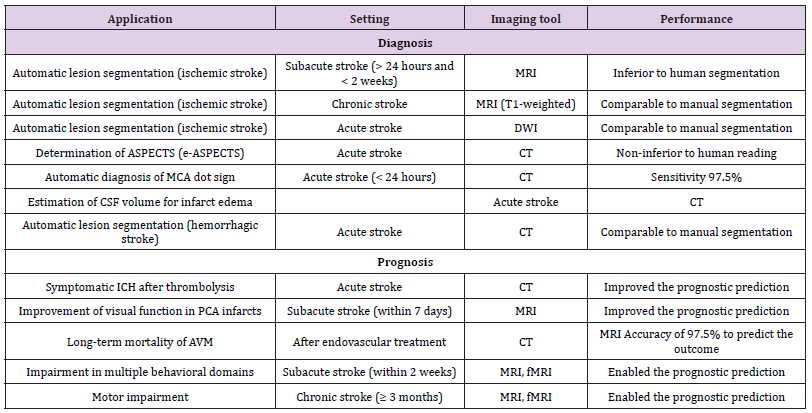
Note: Reproduced from reference 7 under Creative Commons Attribution Non-Commercial License.
Figure 1: The leading 10 diseases considered for artificial intelligence (AI) [6].
Note: Reproduced from reference 6 under Creative Commons Attribution Non-Commercial License.

The neuroimaging techniques like Magnetic Resonance Imaging (MRI) and Computed Tomography (CT) are mostly used in stroke diagnosis. Researchers have used neuroimaging data to develop AI tools to assist stroke diagnosis. Rehme et al. applied an AI method called Support Vector Machines (SVMs) for the classification of “individual motor impairment” after stroke by using resting-state fMRI (functional-MRI) neuroimaging data. The SMV classified patients with hand motor deficits and compared with controls and nonimpaired patients with 83-88% accuracy. The authors highlighted the potential of multivariate fMRI analyses to serve as markers for endophenotypes of functional impairment [8]. AI-based diagnosis by means of CT has also endeavored. The Alberta Stroke Program Early CT Score (ASPECTS) is an established 10-point quantitative topographic CT scan score to assess early ischemic changes. ASPECTS is widely used by neuro-physician to assess the extent of early ischemic changes in brain imaging for acute stroke treatment. It can be assessed through fully automated e-ASPECTS software which is CE (ConformitĂ© EuropĂ©ene, European Conformity) approved commercial ASPECTS scoring tool [9].
Brain lesion segmentation and subsequent quantitative assessment of lesions in medical images provide valuable information for the analysis of brain damage and are vital for the planning of after stroke treatment strategies, monitoring of disease progression and prediction of patient outcome. Kamnitsas et al. have developed Deep Medic software for brain lesion segmentation based on a multi-scale 3D Deep Convolutional Neural Network coupled with a 3D fully connected Conditional Random Field. The Deep Medic has shown excellent performance in challenging lesion segmentation tasks, including traumatic brain injuries, brain tumors, and ischemic stroke lesions [10,11]. RAPID is a neuroimaging software which provides a spontaneous and easily interpretable real-time view of brain perfusion, allowing physicians to determine lesion volumes in ischemic stroke. RAPID analyzes data collected from the CT perfusion scan and quickly provides the stroke team physician with a quantitative value of the volume of brain tissue that is irreversibly damaged and the volume of tissue that can be recovered if blood flow can be restored quickly [12].
A benchmark study applying AI in stroke detection has been reported recently in the journal Nature Medicine [13]. The researchers at the Icahn School of Medicine at Mount Sinai, USA have developed an AI platform to identify a broad range of acute neurological illnesses, such as stroke, hemorrhage, and hydrocephalus and have shown to identify the disease in CT scans in 1.2 seconds, faster than human diagnosis. Eric Oermann, the senior author of the study has clearly mentioned that “with a total processing and interpretation time of 1.2 seconds, such a triage system can alert physicians to a critical finding that may otherwise remain in a queue for minutes to hours [13].
AI-based methods offer several advantages in improving prediction performance for stroke treatment, prognosis, and functional outcome prediction. This helps neuro-physician to identify high-risk patients and guide treatment approaches, leading to decreased morbidity. Several AI-based techniques have been investigated to develop automated platforms for the precise prediction of prognosis and the functional outcome. Park et al. have proposed a Bayesian network model for predicting post-stroke outcomes with available risk factors. They also introduced an online “Yonsei Stroke Outcome Inference System” for predicting functional independence at 3 months and mortality within 1 year in patients with stroke using Bayesian network model [14]. A large number of clinical practice guidelines for IS treatment are available which necessitates the requirement of a unified model for stroke treatment to assist in clinical decision-making process. Therefore, Love et al. have developed a unified treatment model derived through a review of existing clinical practice guidelines, meta-analyses, and clinical trials using a Bayesian belief network [15]. Siegel et al. have developed an AI-based model to predict behavior parameters such as attention, visual memory, verbal memory, language, motor, and visual in stroke patients using resting Functional Connectivity (FC) and lesion topography. It was observed that the visual memory and verbal memory were better predicted by FC, whereas visual and motor impairments were better predicted by lesion topography. The study provides the direct connections between key organizational features of brain networks to brain-behavior relationships in stroke [16]. The MRI is used to capture regional information from brain injury to determine lesion load per region i.e. the proportion of voxels in anatomical structures that are considered to be damaged. Rondina et al. applied a Gaussian Process Regression tool to develop a suitable methodology for predicting long-term motor outcomes from early post-stroke structural MRI [17].
The timely diagnosis of stroke is vital for functional recovery and to minimize mortality. The advancement in imaging technology for stroke diagnosis led to the availability of a large volume of scattered neuroimaging information. Here, AI has been employed in several ways to extract the most coherent information which can be used as an identifier or marker for stroke diagnosis and for analyzing its severity. The ability of AI to provide clinically relevant output information solely depends on the correctness of the input data and the machine learning method used to train the AI model. Therefore, researchers are focusing on the development of a new learning algorithm which can handle a large volume of information and provide more precise output information in a reasonable time frame. As discussed earlier that the timely diagnosis of stroke is the most crucial factor for the patient’s recovery from stroke, the AIbased solutions provide rapid and precise information to assist the physician in decision making.
Potential Carcinogenic Risk Evaluation of Pb in Selected Canned Foods Obtained from Superstores in Nigeria-https://biomedres01.blogspot.com/2020/12/potential-carcinogenic-risk-evaluation.html
More BJSTR Articles : https://biomedres01.blogspot.com
No comments:
Post a Comment
Note: Only a member of this blog may post a comment.